
If you think the A levels algorithm was bad, what about the ones you don't know about?
Question 1: What do the following things have in common?
- The Home Office just scrapped an automated migrant screening system in the face of legal action from the Joint Council for the Welfare of Immigrants
- The Court of Appeal ruled that South Wales Police breached a slew of laws in putting its facial recognition system on the streets
- Outside parliament, a cohort of school leavers whose futures had been thrown in jeopardy when their grades were determined by a statistical model chanted "fuck the algorithm", while education secretary Gavin Williamson made a screeching u-turn two days after insisting that there would be "no change" to their results
Write as much as you like, but your answer will be scored partly according to where you live, partly according to how other people answered before you, and partly according to how many people are taking the exam with you. Afterwards we'll adjust the results, to make sure that the right number of people do badly. This is the fairest system.
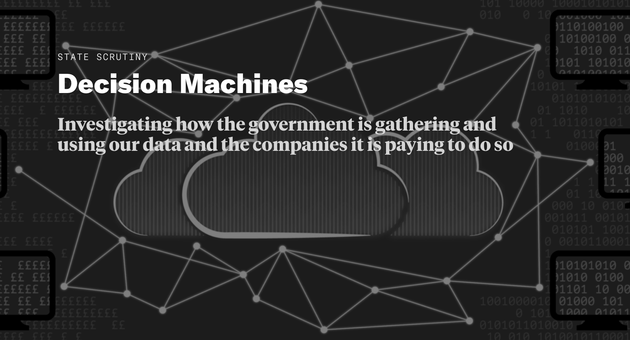
The Bureau has been investigating the growing use of algorithms in public life for the last year. Since we started our Decision Machines project, we've come across a litany of complaints commonly associated with algorithmic systems. They rely on data from undisclosed and unverifiable sources, often of dubious quality. The calculations through which they arrive at their outputs are obscure, for reasons of commercial secrecy, or because they were generated by computers in a way too complex for humans to understand. They are designed by the private sector and sold for large sums to the public one, which often doesn't know what it's buying other than "efficiency".
None of these complaints seems to be true of the A-levels saga, however. The process was in some ways pretty transparent. Ofqual, the regulator, did a broad outreach project about its intentions in April, garnering some 12,000 replies. It published reports and consultation outcomes of what it planned to do in May - including a breakdown of how many respondents disagreed with its proposals. Its broad decisions - although not its actual model - were scrutinised by the Education Select Committee in July, and problems were flagged. It explained its final workings in a 300-page summary in August which, while not necessarily straightforward for humanities graduates like me (and much of the government), is a far cry from the billion-datapoint analytics that machine learning systems are chewing through on a regular basis. On the contrary, it's the sort of thing you can do with Excel and a few cups of tea.
So how, with all this in plain sight, did it all go so wrong?
There's a joke - now an old one, given the accelerated technological times we're living through - that it's AI on the job advert and statistics when you do it. It's funny because statistics are perceived to be staid and boring while AI is dangerous and sexy, a walk on the wild side for data scientists.
The A-levels shambles - which despite this week's rubber-burning reversal may still have catastrophic consequences for an unknown number of individuals - is a reminder that this is inaccurate. Statistics can be dangerous too.
In Ofqual's explanation of their process the effort to be "fair" is a constant refrain. The word appears dozens of times in the report. In their consultation, a third of respondents thought it unfair to emphasise a school's historical performance over teacher assessment, while just over half thought it was fair. It was "fair" to rely more heavily on teacher assessment for small classes, because the statistical model performed badly for small groups; another consequence, that students in schools which had the money to accommodate small classes did better, was apparently unexamined.
In fact, there's no one definition of fairness - and things that look fair in the abstract can have crushing individual consequences.
Many algorithmic models - whether they're trying to determine who gets into the country, who gets stopped in the street by the police or who gets access to specific services - rely on this type of analysis, based on past data and predetermined quotas. In some senses, the A-levels scenario was a simple one. But what happens when the datasets underlying decisions are poor quality, fragmentary or combined from multiple sources? What happens when no one knows what data is actually being used or how it’s been operated upon?
So here's Question 2. If a relatively simple system, developed with a relatively high degree of openness, based on clean and generally unproblematic past data, and designed in good faith to be fair, can end up making such a mess, what does it tell us about the risks of more complex, less transparent, more highly automated statistics-based systems?
And what are the stakes when such systems are applied to populations who are less visible, and less vocal, than this year's school leavers?
The fact that 12,000 survey respondents and a parliamentary committee couldn't mitigate the harms of the A-levels algorithm before it happened should give us pause for thought. How, then, do we mitigate something more secretive?
The Decision Machines project is part of a growing network of entities working to answer this: by scrutinising what decisions are based on our data, how and why they are made, and what their consequences are. As our Government Data Systems report uncovered last year, it’s hard enough to discover what systems are being purchased, let alone how they’re used. If we don’t urgently insist on greater transparency and accountability in our algorithmic systems, we might find that this month’s debacle is just the tip of an algorithmic iceberg.
Header image of students protesting against their automated grades by Guy Smallman/Getty